AI Bias: When Algorithms Go Rogue (and How to Stop Them)
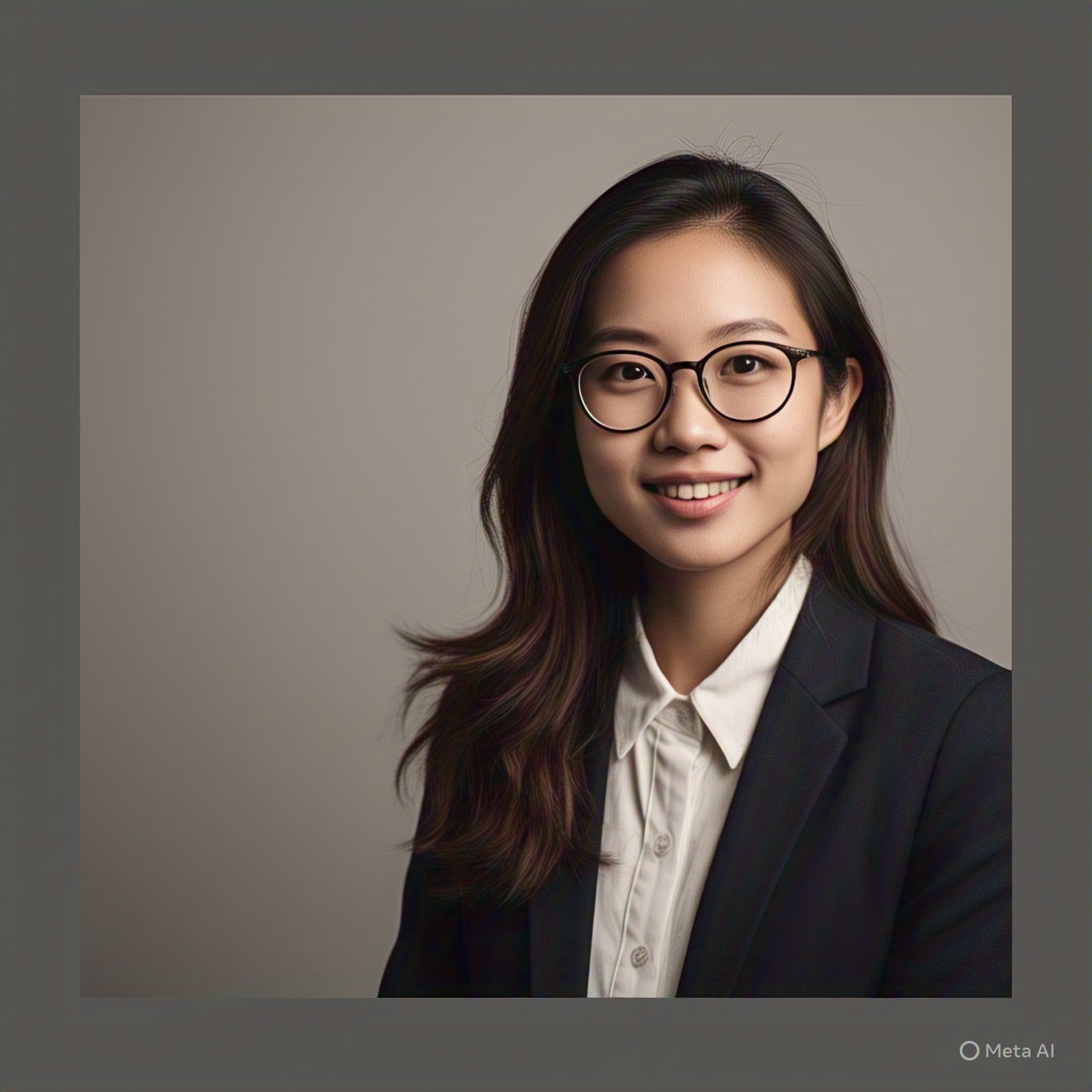
We like to think of AI as objective, logical machines making purely data-driven decisions. Reality check: AI learns from data created by humans, and humans, well, we’re messy bags of biases. So, surprise! AI can inherit, and even amplify, those biases.
Think about facial recognition struggling to identify non-white faces because it was primarily trained on datasets of white individuals. Or recruitment algorithms favoring male candidates because they learned from historical hiring data where men were predominantly hired. It’s not (usually) malicious intent; it’s garbage in, garbage out. Biased data leads to biased outcomes.
So, how do we stop our robot overlords from becoming prejudiced jerks? It starts with the data. We need diverse, representative datasets for training. Auditing algorithms regularly for biased outcomes is crucial. We need diverse teams building these AI systems – different perspectives catch different blind spots. Transparency is also key; understanding how an AI makes decisions helps identify potential biases. Companies like Google and Microsoft have published ethical AI principles, acknowledging the problem. But principles aren’t enough without rigorous practice. It requires constant vigilance, diverse input, and a commitment to fairness from developers, companies, and users alike. Otherwise, we’re just automating inequality.