AI in Healthcare: Beyond the Hype
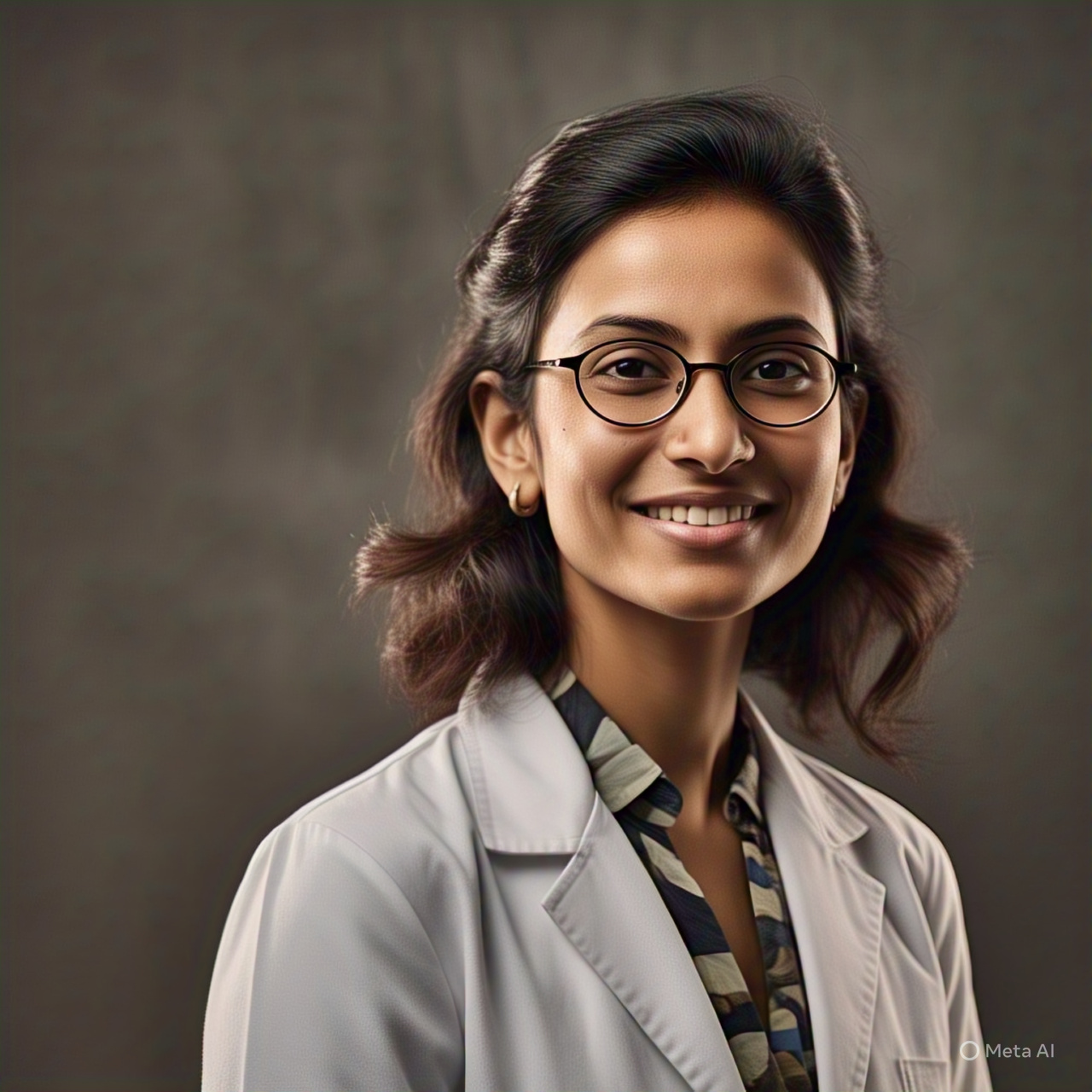
Between tech CEOs promising to “solve healthcare” and sci-fi scenarios of robot doctors, it’s hard to separate AI healthcare reality from Silicon Valley fantasy. So let’s cut through the noise and look at where AI is actually making a difference—and where it’s still struggling in scrubs.
Radiology was among the first battlegrounds. AI systems can now detect certain abnormalities in medical images with accuracy rivaling specialists. At Massachusetts General Hospital, an AI system flagged a small brain aneurysm that had been missed on previous reads, potentially saving the patient’s life. But these systems aren’t replacing radiologists—they’re working alongside them, serving as highly specialized second opinions.
Administrative tasks—healthcare’s paperwork purgatory—are seeing genuine transformation. A midsize hospital system I worked with implemented AI for patient scheduling and documentation. Their physicians went from spending 2 hours daily on paperwork to just 25 minutes. That’s not just efficiency; it’s a mental health intervention for burnt-out clinicians.
Drug discovery might be the most promising frontier. Traditional development takes years and billions, but AI is accelerating the process dramatically. Insilico Medicine identified a novel treatment candidate for idiopathic pulmonary fibrosis in just 18 months versus the typical 3-5 years. The compound is now in clinical trials—remarkable speed for an industry where progress is typically measured in decades.
But healthcare isn’t an optimization problem that can simply be coded away. A major health system implemented an AI system for predicting patient deterioration, only to find nurses largely ignoring its alerts. The reason? The system didn’t account for the nurses’ existing workflows and cognitive load. Technology deployment without human-centered design is just expensive digital decoration.
Data quality remains the elephant in the examination room. Even sophisticated algorithms can’t overcome the foundational issues in healthcare data—inconsistent recording practices, biased historical treatments, and fragmented records. An algorithm trained on predominantly white male patients will struggle with diverse populations, potentially exacerbating existing healthcare disparities.
The most successful AI healthcare implementations aren’t flashy. They’re thoughtfully integrated tools that respect clinical expertise while addressing concrete problems. As the physician-informaticist at my local hospital puts it: “I don’t need AI to practice medicine for me. I need it to handle everything that keeps me from practicing medicine.”